Big data challenges: Tackling 5 bear-traps in learning analytics (and how to avoid them)
Research over the past few years has shown that while learning analytics and data are seen as a critical focus area for learning and development, learning professionals are not confident in their data skills and see big data challenges ahead as they respond to calls from the business to be more data-driven. Where are the potential pitfalls for L&D, and how should they avoid them?
When it comes to data, L&D often seems to suffer from low self-esteem. Perhaps it’s not so surprising when you consider some of the things they hear from the conference platform: ‘every department in the organization has better data than you’ … ‘you have more data in your Fitbit than you have about your learners!’.
However, perhaps learning professionals really shouldn’t beat themselves up too much. Consider the scale of the task they are facing. Learning is a hard thing to observe in practice, let alone measure. It’s much tougher to measure than marketing, for instance (there’s no ‘buy’ button on a learning program). It also takes time, and happens against the background of today’s fast-changing, often chaotic business environment, where nobody has time to spare. When a person’s performance improves, it is often difficult to disentangle the part learning played in that success from other factors such as incentivization or things happening in their personal life.
For these reasons, learning analytics is complicated. It’s a whole field on its own of academic study, and one of fairly recent origin at that, just over a decade old. In addition, the training world has amassed over the last sixty years numberless refinements to, and revisions of, its own popular four-step model for learning evaluation created by Kirkpatrick and Katzell. So there’s a lot to know.
Of course, you don’t need to know all of it to start making practical use of learning data any more than you need a degree in mechanical engineering to drive a car. But there are some notable pitfalls to avoid when you’re on the path to greater data literacy; bear-traps that often claim the unwary traveller. Here are five – and how to avoid them.
5 Traps in Learning Analytics
1. Taking too narrow a view of the subject
People often box themselves in by taking a very limited view of what can be done with learning data and thinking of it solely in terms of historical evaluation of past training programs.
To avoid this pitfall we think you should take a 360 approach to learning data. You wouldn’t drive using only your rear-view mirror, so don’t restrict your focus to learning evaluation (important as that is): a more holistic approach will give you also side mirrors, dash-cam and a clearer view of the road ahead. It’s not all about evaluation or analytics.
So here’s a better box for your thinking: a handy data schema given by Donald Clark in his book ‘Artificial Intelligence for Learning’ that will help you appreciate the full scope and opportunity offered by taking on big data challenges. The schema is based on goals, because as Clark writes, “it is almost pointless to gather data if it remains unloved and unused. There must be a purpose to the endeavor.”
Goal | Example |
Describe | Tracking and visualization of learning data |
Analyze | Evaluation, linking to business performance and improve training ROI, determining how people learn best |
Predict | Predict performance and whether people are likely to drop out |
Prescribe | Recommendation engines, adaptive learning |
2. Being too reactive
If you wait to be asked for better data by the business, there is a theory called the ‘conspiracy of convenience’ that suggests it might never happen. This theory was advanced by analyst David Wilson and learning guru Charles Jennings, and it maintains that learning departments and their internal clients collude in a largely unspoken way to avoid the subject of learning impact. The client doesn’t ask for data to determine whether a learning intervention had any impact on performance, and the learning department doesn’t volunteer to supply it.
However, if you spot this happening in your organization and you go along with it, you will run the risk of irrelevance and ultimately obsolescence. So be proactive. Start today in making data a central part of your practice, and educate your internal and external customers on the benefits of taking a data-led approach.
3. Getting in a rut with data sources
Many learning people fixate on particular data sources that is close to hand and relatively easy to gather – e.g. course completions and test scores from your learning management system – and fail to look further afield. There is an ever-increasing amount of data available now from all sorts of sources. The danger of sticking to limited sources is that it’s rare to make an easy causal link between one data source on learning and an improvement in performance. In other words, it’s hard to find a ‘smoking gun’ when it comes to evaluating impact.
Solution? Use multiple data sources to evaluate and take a ‘portfolio of evidence approach’. Chances are you will only be able to prove that your intervention might be the reason something changed; but you can stack that deck in your favor with more data points.
4. Siloed thinking
It can be all too tempting to ‘stay within your lane’ and ignore what is going on in the rest of the organization. However, learning doesn’t happen in a vacuum. Learners are primarily employees with day-to-day work that takes up a lot more of their time than their learning activities, so it follows that the vast majority of data about their performance, skills gaps and knowledge needs are not in the learning management system but in other parts of the organization.
Today’s organizations are awash with data. Starting from where you are, use the data resources that are on hand in other parts of the business, though it might mean forging new alliances and learning new skills to get hold of it.
5. Bunker mentality
Given all the demands and stresses of dealing with the business, it is all too easy for learning professionals to feel undervalued and misunderstood, and to start to adopt a bunker mentality. The defensive mindset this promotes is the very worst thing that can happen in a situation where the learning function is needing to open up and learn new skills to deal with the big data challenges. Showing a gap in knowledge and skills of any kind can run the risk of being perceived as a weakness. But you can’t be a learning professional and not have the courage to learn yourself!
To combat bunker mentality adopt an agile mindset. Start small, fail fast, learn by doing. Learning Pool has valuable free tools available to help you, all available at the link below: a maturity model to assess where you are in your data journey, and the Learning Analytics Canvas to help you plan for impact studies.
Download our new eBook, ‘Adding data and learning analytics to your organization’ to find out more.
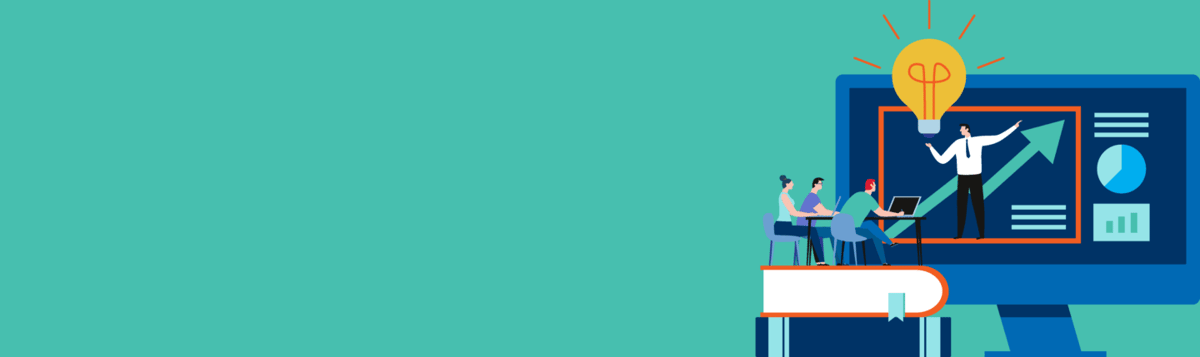
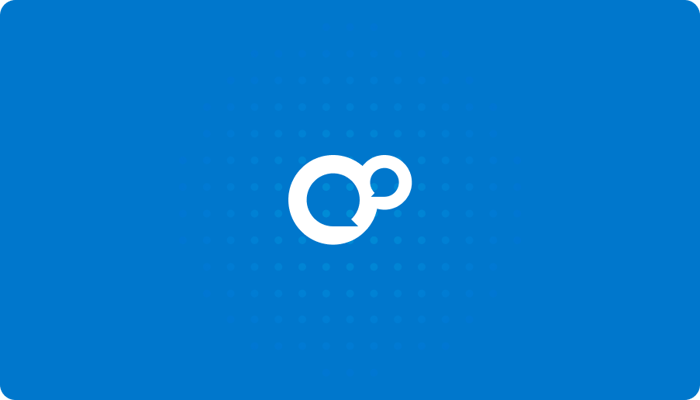
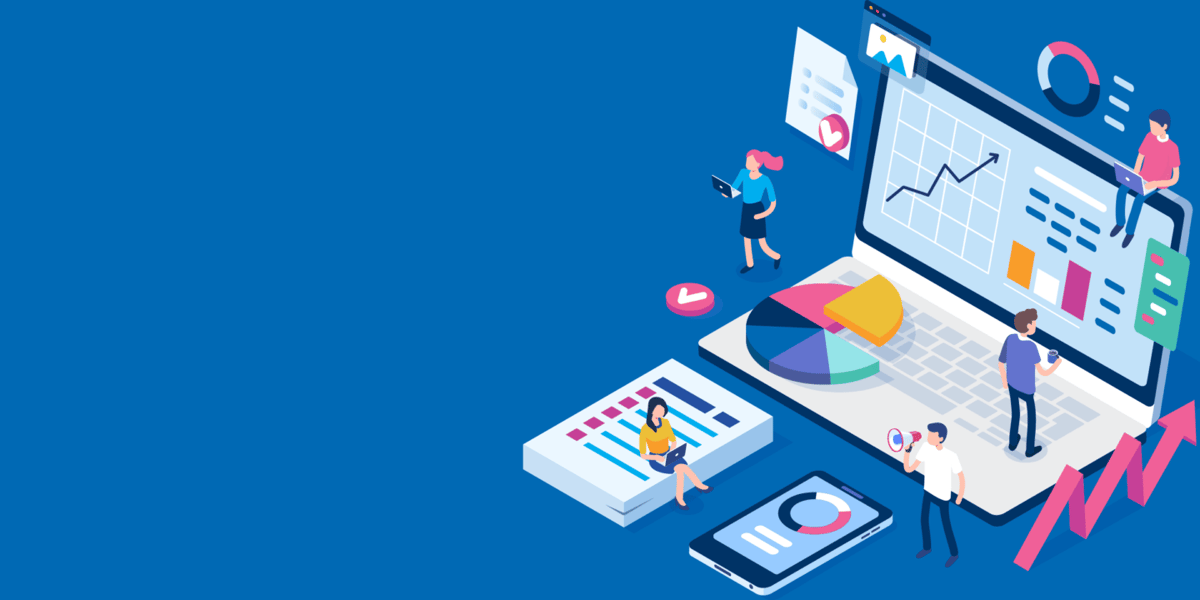